What is Human in the Loop AI?
- Sophia P
- Sep 5, 2024
- 6 min read
Human-in-the-loop (HITL) is a collaborative approach that brings human input and expertise into the machine learning (ML) and artificial intelligence (AI) process. Simply put - getting humans involved in stages of artificial intelligence. That's the idea behind "human-in-the-loop" or HITL for short. Instead of letting machines learn entirely on their own, HITL is a way for humans and AI to work together. People provide guidance, input and expertise to improve how machines learn. Not dissimilar to a senior (human) employee providing guidance to a junior employee.
The smartest engineers, data scientists and subject matter experts team up with machine algorithms to build better machine learning (ML) systems through interactive learning. The models crunch huge volumes of data but when they hit a snag (low confidence result), humans can step in, correct the output, and ultimately enhance the model.
The goal is combining the scaled analytical strengths of AI with human judgement, common sense and creativity. Supporters say this mixed approach leads to robust models that reduce unintended biases. This is not to say that HITL is sufficient on its own, as potential for hallucinations, data drift, and other risks remain valid concerns even with this approach. However, by keeping people involved, HITL systems can mitigate many of these risks and adapt more safely to new situations down the line compared to pure automated learning.

AI with Human Expertise in the Loop Benefits
The marriage of people and artificial intelligence has many benefits:
Accuracy
Human oversight and human feedback validate and correct AI decisions, across applications from healthcare to autonomous driving. In content moderation, AI can miss content that should be flagged (a false negative) and flag content that’s harmless (a false positive). Humans are crucial in content moderation as they can interpret context, multilingual text and cultural, regional and socio-political nuances of local markets.
Ethical Compliance
Humans keep AI in check of societal and ethical boundaries.
Accountability
With humans involved, decision trails are more transparent, more accountable.
Flexibility
Allows AI to adapt to real-world complexities where human intuition and judgment matters.
Transparency and Explainability
Enhances transparency and explainability for ML models (still short of proper Explainable AI - or XAI - more on that to follow) by giving insights into the decision making process. Human factors in the design of HITL systems is key to improving user experience and system effectiveness.
Data Collection
Machine learning models need large amounts of training data to be successful. In situations where large datasets are not available, the algorithm will produce unreliable results. Having a human-in-the-loop helps to create data and ensure accuracy, plays a big role in active learning.
Removing Bias
AI programs built by humans and based on historical data will perpetuate inequalities. Having a human-in-the-loop can detect and correct bias early.
Saving Time
Machine intelligence can save a lot of time and cost by filtering and reducing large amount of data. The task can then be handed over to humans for final review. While the whole process can’t be automated, a big part of it can, saving time.
Interactive Machine Learning with Human in the Loop Examples
Autonomous Vehicles
Role of Human Intervention: In autonomous driving, AI does the routine and decision making. But human drivers are needed for oversight and to take control in emergency or complex traffic scenarios. This combination ensures safety and reliability, leveraging AI’s efficiency while mitigating risks through human intervention.
Healthcare Diagnostics
Role of Human Intervention: AI analyzes medical images like X-rays and MRIs to identify potential anomalies. Human doctors then review the AI generated insights to ensure the final diagnosis is accurate. Data scientists play a key role in refining the AI generated insights to improve the collaboration between AI and human expertise to improve diagnostic accuracy and patient care.
Customer Service Chatbots
Role of Human Intervention: Deep learning technologies are used to build AI chatbots to handle simple customer queries. For complex or sensitive issues, the chatbot transfers the conversation to a human agent. So simple questions are answered quickly and complex problems get the human touch.
Content Moderation on Social Media
Role of Human Intervention: AI systems flag potential harmful or inappropriate content on social media platforms. Human moderators then review the flagged items to make the final call, balancing automated efficiency with human judgment to ensure fair and ethical content moderation.
Marketing Analytics and Customer Insights
Role of Human Intervention: Marketing companies use AI to analyze large datasets and extract insights about customer behavior and preferences. Humans review the AI generated insights to ensure they are accurate and relevant before making marketing decisions. For example, while AI can predict trends and segment audiences, marketing professionals tailor campaigns based on human understanding and context.
Financial Trading and Investment Advisory
Role of Human Intervention: AI systems analyze market data and suggest trades or investment opportunities. Machine learning algorithms are used to handle complex data and improve the accuracy of the analysis. Traders and financial advisors then review the AI recommendations to account for market sentiments, regulatory changes and other factors AI might not fully understand. This collaboration helps in making more informed and balanced investment decisions.
What Does This Mean for Your Business?
As businesses are rapidly adopting AI, even with Human-in-the-Loop (HITL) methodologies, human knowledge is a key component in building AI systems. And the importance of human interaction in refining AI business solutions can’t be overstated. The key benefits of a HITL approach include:
AI systems operate within regulatory and ethical boundaries.
Sensitive data and data integrity are protected.
AI is transparent and explainable to build trust with stakeholders.
Employees have time to periodically monitor AI use and improve processes.
AI Guardian's AI governance platform helps you implement a Human-in-the-Loop approach and ensure your AI systems are secure, compliant and performing optimally. AI Guardian helps disseminate your AI policies to employees, tracks AI projects and assess risk to guide human intervention and provides a dashboard for human oversight of AI activity.
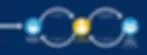
Getting Real With Humans and AI
Personally, I think of AI as a new employee in my company. As a leader, I want my employees to have autonomy and confidence. Initially, they may need more oversight and education to ensure they understand their tasks and are doing them correctly. As team members grow, they have greater confidence in more of their work as well as a better understanding of where their confidence is lower and some guidance would help get them in the right direction. Like a new hire, an AI system may need closer monitoring and more human intervention when it’s first deployed. With regular reviews and adjustments, the AI can be fine tuned to make sure it’s aligned with company goals and standards.
Active learning (AL) is key in this scenario where the AI model iteratively asks human experts to label uncertain data points. This approach improves model performance while minimizing the labeling effort by selecting the right data points to be annotated.
As employees get more proficient in their roles, the frequency of reviews may decrease. Trust in their capabilities grows and they need less hands on guidance – though periodic reviews are still necessary to maintain quality and address any new issues. This is the lifecycle of AI in a human-in-the-loop framework.
Initially AI systems need a lot of human input to learn and adapt. This stage involves close human oversight, constant feedback and iterative improvements. As the AI gets more accurate and reliable the level of supervision can be reduced. But even as the AI becomes more autonomous it still needs periodic human reviews to make sure it’s operating within the desired boundaries and adapting to new challenges or changes in the environment.
This is a great example of the harmony between human intelligence and machine efficiency. Managers, like supervisors of AI, need to be adaptable in their oversight roles, to provide guidance or step back based on performance and requirements. Ultimately, this collaborative approach - done properly with quality feedback/input and other effective Responsible AI Governance measures in place - can help AI systems work more effectively and mitigate certain risks.
Human in the Loop AI with Human Feedback
Human-in-the-loop machine learning is a powerful match between human intuition and AI.
With HITL, companies and developers can build more effective, accurate and ethical AI across many areas like natural language processing, computer vision and speech recognition.
As HITL use evolves and grows, the interactive system will become more and more important in building smarter and more reliable AI, driving more innovative AI advancements with greater societal benefit and reduced risk.
Reflections on Human in the Loop AI
It bears repeating, however, that - while HITL approaches offer great benefits - they remain limited by the quality of the human input being provided and are by no means sufficient on their own. HITL may be a helpful part of an overall strategy, but it is just that - a piece of a larger puzzle. A comprehensive AI Governance approach - which may consider Human-in-the-Loop as one component technique along with appropriate guidelines for the human workforce and guardrails for the machine models - remains the most effective means of ensuring accurate, responsible AI.
By Bart Layton, CEO - AI Guardian
Fact Checked by Robin Hackney